

We perform numerical simulations and compare the proposed method with existing gradient-free and gradient-based optimization algorithms.
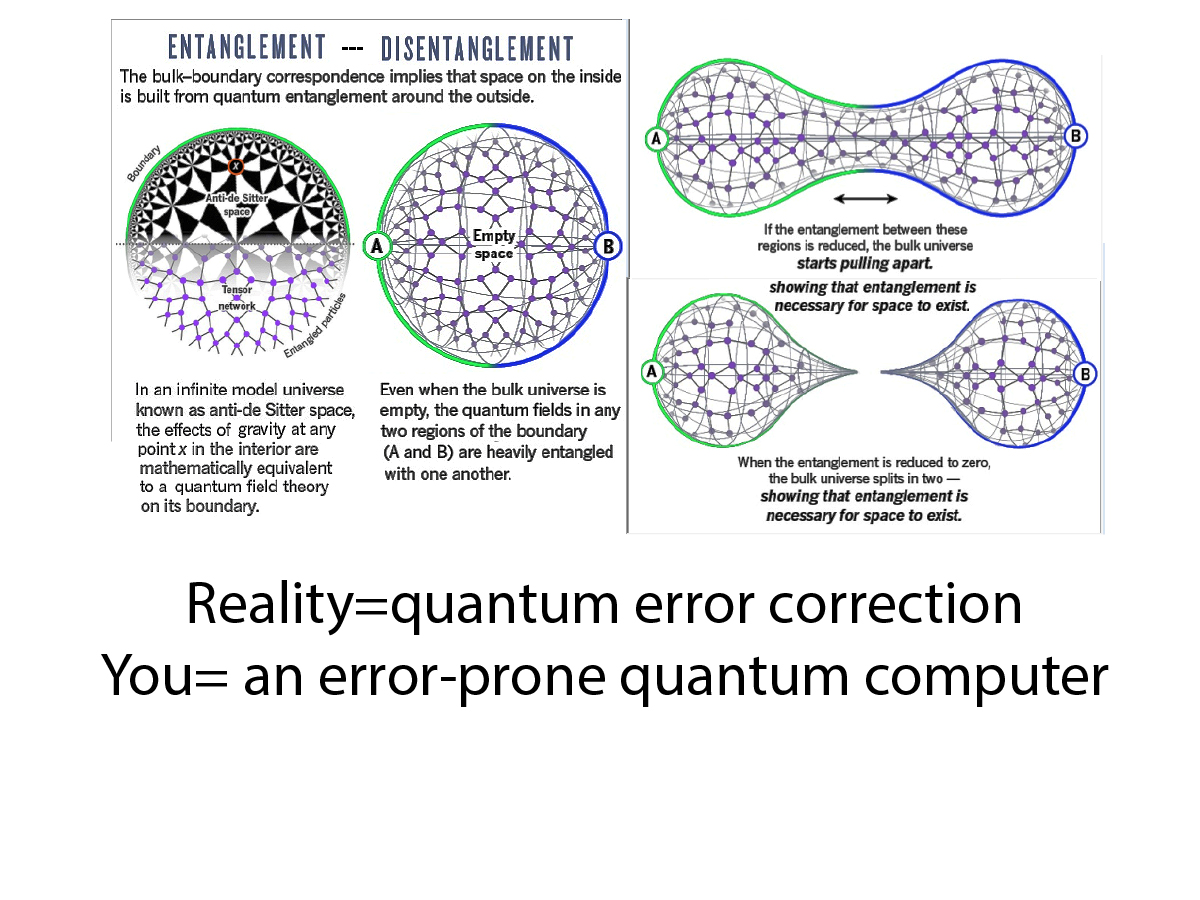
By repeatedly performing this procedure, we can optimize the parameterized quantum circuits so that the cost function becomes as small as possible. Furthermore, even in general cases, the cost function is given by a simple sum of trigonometric functions with certain periods and hence can be minimized by using a classical computer. In fact, if we choose a single parameter, the cost function becomes a simple sine curve with period 2π, and hence we can exactly minimize with respect to the chosen parameter.

Specifically, the optimization problem of the parameterized quantum circuits is divided into solvable subproblems by considering only a subset of the parameters. We propose a sequential minimal optimization method for quantum-classical hybrid algorithms, which converges faster, robust against statistical error, and hyperparameter-free.
MACHINE LEARNING QUANTUM ERROR CORRECTION FULL
While in principle this framework can be instantiated with environments modelling circuit level noise, we take a first step towards this goal by using deepQ learning to obtain decoding agents for a variety of simplified phenomenological noise models, which yield faulty syndrome measurements without including the propagation of errors which arise in full circuit level noise models.
MACHINE LEARNING QUANTUM ERROR CORRECTION CODE
In this work, we show that the problem of decoding such codes can be naturally reformulated as a process of repeated interactions between a decoding agent and a code environment, to which the machinery of reinforcement learning can be applied to obtain decoding agents. As such, obtaining fast and flexible decoding algorithms for these codes, within the experimentally realistic and challenging context of faulty syndrome measurements, without requiring any final read-out of the physical qubits, is of critical importance. Topological error correcting codes, and particularly the surface code, currently provide the most feasible road-map towards large-scale fault-tolerant quantum computation. Additionally, it generates logical gates not found in the current literature for the ] code, the ] code, and the ] code. We test the procedure by simulation on classical computers on small quantum codes of four qubits to fifteen qubits and show that it finds most logical gates known in the current literature. It enables automatic discovery of logical gates from analytically designed error correcting codes and can be extended to error correcting codes found by numerical optimizations. This procedure can be implemented on near-term quantum computers via quantum process tomography. Our technique is to use variational circuits for learning both the logical gates and the physical operations implementing them. We present an automated procedure which generates logical operations given known encoding and correcting procedures. Here we study the problem of designing logical operations for quantum error correcting codes. Quantum error correcting codes protect quantum computation from errors caused by decoherence and other noise.
